Learning non-linear probabilistic computations in recurrent spiking networks
Lefebvre, Jérémie;
Black, Michael
(2013)
mla clipboard |
Lefebvre J., et al. "Learning non-linear probabilistic computations in recurrent spiking networks.", timms video, Universität Tübingen (2013): https://timms.uni-tuebingen.de:443/tp/UT_20130926_009_bestcon_0001. Accessed 02 Apr 2025. |
apa clipboard |
Lefebvre, J. & Black, M. (2013). Learning non-linear probabilistic computations in recurrent spiking networks. timms video: Universität Tübingen. Retrieved April 02, 2025 from the World Wide Web https://timms.uni-tuebingen.de:443/tp/UT_20130926_009_bestcon_0001 |
harvard clipboard |
Lefebvre, J. and Black, M. (2013). Learning non-linear probabilistic computations in recurrent spiking networks [Online video]. 26 September. Available at: https://timms.uni-tuebingen.de:443/tp/UT_20130926_009_bestcon_0001 (Accessed: 2 April 2025). |
file download | bibtex endnote |
Information
current
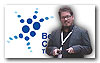
00:14:31
Learning non-linear probabilistic computations in recurrent spiking networks
(2013-09-26)
related
Rapid developmental changes in the patterns of population activity accompany the onset of visual experience in ferret visual cortex
(2013-09-27)
Structural Determinants of Spatial Representations in Layer 2 of Medial Entorhinal Cortex
(2013-09-27)
Stimulus fluctuations together with top-down feedback can account for the dynamics of Choice Probability in MT
(2013-09-27)