Machine Learning in Econometrics
(44 Einträge)
Lecture Machine Learning in Econometrics, 1. Lesson
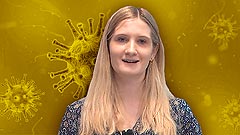

Title: | Lecture Machine Learning in Econometrics, 1. Lesson |
Description: | Vorlesung im SoSe 2021; Freitag, 30. April 2021 |
Creator: | Jantje Sönksen (author) |
Contributor: | ZDV Universität Tübingen (producer) |
Publisher: | ZDV Universität Tübingen |
Date Created: | 2021-04-30 |
Subjects: | Wirtschaftswissenschaft, Machine Learning, Econometrics, |
Identifier: | UT_20210430_001_sose21mleco_0001 |
Rights: | Rechtshinweise |
Abstracts: | This module illustrates how machine learning techniques can be exploited in economic research and applications. It offers a thorough analysis of a variety of tools in machine learning and links them to econometric analysis. The class focuses on supervised machine learning algorithms such as: decision trees, (logistic) regressions, naïve Bayes, nearest neighbor, neural networks, and support vector machines. The lecture also covers feature selection and hyper-parameter tuning methods. A practical PC-Lab class is an essential part of the module. Students apply state-of-the-art machine learning techniques and understand how these are linked to standard econometrics. They command different machine learning methods and apply them to economic problems. They are aware of the respective advantages and shortcomings of these methods and discuss their results critically. |
Lecture Machine Learning in Econometrics, 2. Lesson
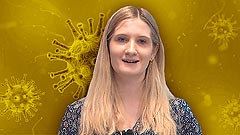

Title: | Lecture Machine Learning in Econometrics, 2. Lesson |
Description: | Vorlesung im SoSe 2021; Freitag, 30. April 2021 |
Creator: | Jantje Sönksen (author) |
Contributor: | ZDV Universität Tübingen (producer) |
Publisher: | ZDV Universität Tübingen |
Date Created: | 2021-04-30 |
Subjects: | Wirtschaftswissenschaft, Machine Learning, Econometrics, |
Identifier: | UT_20210430_002_sose21mleco_0001 |
Rights: | Rechtshinweise |
Abstracts: | This module illustrates how machine learning techniques can be exploited in economic research and applications. It offers a thorough analysis of a variety of tools in machine learning and links them to econometric analysis. The class focuses on supervised machine learning algorithms such as: decision trees, (logistic) regressions, naïve Bayes, nearest neighbor, neural networks, and support vector machines. The lecture also covers feature selection and hyper-parameter tuning methods. A practical PC-Lab class is an essential part of the module. Students apply state-of-the-art machine learning techniques and understand how these are linked to standard econometrics. They command different machine learning methods and apply them to economic problems. They are aware of the respective advantages and shortcomings of these methods and discuss their results critically. |
Lecture Machine Learning in Econometrics, 3. Lesson
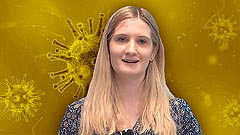

Title: | Lecture Machine Learning in Econometrics, 3. Lesson |
Description: | Vorlesung im SoSe 2021; Freitag, 30. April 2021 |
Creator: | Jantje Sönksen (author) |
Contributor: | ZDV Universität Tübingen (producer) |
Publisher: | ZDV Universität Tübingen |
Date Created: | 2021-04-30 |
Subjects: | Wirtschaftswissenschaft, Machine Learning, Econometrics, |
Identifier: | UT_20210430_003_sose21mleco_0001 |
Rights: | Rechtshinweise |
Abstracts: | This module illustrates how machine learning techniques can be exploited in economic research and applications. It offers a thorough analysis of a variety of tools in machine learning and links them to econometric analysis. The class focuses on supervised machine learning algorithms such as: decision trees, (logistic) regressions, naïve Bayes, nearest neighbor, neural networks, and support vector machines. The lecture also covers feature selection and hyper-parameter tuning methods. A practical PC-Lab class is an essential part of the module. Students apply state-of-the-art machine learning techniques and understand how these are linked to standard econometrics. They command different machine learning methods and apply them to economic problems. They are aware of the respective advantages and shortcomings of these methods and discuss their results critically. |
Lecture Machine Learning in Econometrics, 4. Lesson
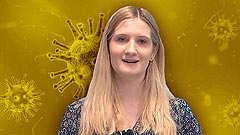

Title: | Lecture Machine Learning in Econometrics, 4. Lesson |
Description: | Vorlesung im SoSe 2021; Freitag, 30. April 2021 |
Creator: | Jantje Sönksen (author) |
Contributor: | ZDV Universität Tübingen (producer) |
Publisher: | ZDV Universität Tübingen |
Date Created: | 2021-04-30 |
Subjects: | Wirtschaftswissenschaft, Machine Learning, Econometrics, |
Identifier: | UT_20210430_004_sose21mleco_0001 |
Rights: | Rechtshinweise |
Abstracts: | This module illustrates how machine learning techniques can be exploited in economic research and applications. It offers a thorough analysis of a variety of tools in machine learning and links them to econometric analysis. The class focuses on supervised machine learning algorithms such as: decision trees, (logistic) regressions, naïve Bayes, nearest neighbor, neural networks, and support vector machines. The lecture also covers feature selection and hyper-parameter tuning methods. A practical PC-Lab class is an essential part of the module. Students apply state-of-the-art machine learning techniques and understand how these are linked to standard econometrics. They command different machine learning methods and apply them to economic problems. They are aware of the respective advantages and shortcomings of these methods and discuss their results critically. |
Lecture Machine Learning in Econometrics, 5. Lesson
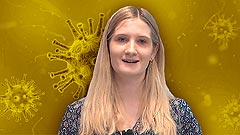

Title: | Lecture Machine Learning in Econometrics, 5. Lesson |
Description: | Vorlesung im SoSe 2021; Freitag, 07. Mai 2021 |
Creator: | Jantje Sönksen (author) |
Contributor: | ZDV Universität Tübingen (producer) |
Publisher: | ZDV Universität Tübingen |
Date Created: | 2021-05-07 |
Subjects: | Wirtschaftswissenschaft, Machine Learning, Econometrics, |
Identifier: | UT_20210507_001_sose21mleco_0001 |
Rights: | Rechtshinweise |
Abstracts: | This module illustrates how machine learning techniques can be exploited in economic research and applications. It offers a thorough analysis of a variety of tools in machine learning and links them to econometric analysis. The class focuses on supervised machine learning algorithms such as: decision trees, (logistic) regressions, naïve Bayes, nearest neighbor, neural networks, and support vector machines. The lecture also covers feature selection and hyper-parameter tuning methods. A practical PC-Lab class is an essential part of the module. Students apply state-of-the-art machine learning techniques and understand how these are linked to standard econometrics. They command different machine learning methods and apply them to economic problems. They are aware of the respective advantages and shortcomings of these methods and discuss their results critically. |
Lecture Machine Learning in Econometrics, 6. Lesson
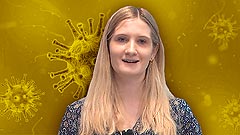

Title: | Lecture Machine Learning in Econometrics, 6. Lesson |
Description: | Vorlesung im SoSe 2021; Freitag, 07. Mai 2021 |
Creator: | Jantje Sönksen (author) |
Contributor: | ZDV Universität Tübingen (producer) |
Publisher: | ZDV Universität Tübingen |
Date Created: | 2021-05-07 |
Subjects: | Wirtschaftswissenschaft, Machine Learning, Econometrics, |
Identifier: | UT_20210507_002_sose21mleco_0001 |
Rights: | Rechtshinweise |
Abstracts: | This module illustrates how machine learning techniques can be exploited in economic research and applications. It offers a thorough analysis of a variety of tools in machine learning and links them to econometric analysis. The class focuses on supervised machine learning algorithms such as: decision trees, (logistic) regressions, naïve Bayes, nearest neighbor, neural networks, and support vector machines. The lecture also covers feature selection and hyper-parameter tuning methods. A practical PC-Lab class is an essential part of the module. Students apply state-of-the-art machine learning techniques and understand how these are linked to standard econometrics. They command different machine learning methods and apply them to economic problems. They are aware of the respective advantages and shortcomings of these methods and discuss their results critically. |
Lecture Machine Learning in Econometrics, 7. Lesson
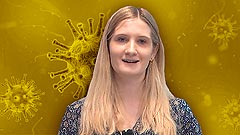

Title: | Lecture Machine Learning in Econometrics, 7. Lesson |
Description: | Vorlesung im SoSe 2021; Freitag, 07. Mai 2021 |
Creator: | Jantje Sönksen (author) |
Contributor: | ZDV Universität Tübingen (producer) |
Publisher: | ZDV Universität Tübingen |
Date Created: | 2021-05-07 |
Subjects: | Wirtschaftswissenschaft, Machine Learning, Econometrics, |
Identifier: | UT_20210507_003_sose21mleco_0001 |
Rights: | Rechtshinweise |
Abstracts: | This module illustrates how machine learning techniques can be exploited in economic research and applications. It offers a thorough analysis of a variety of tools in machine learning and links them to econometric analysis. The class focuses on supervised machine learning algorithms such as: decision trees, (logistic) regressions, naïve Bayes, nearest neighbor, neural networks, and support vector machines. The lecture also covers feature selection and hyper-parameter tuning methods. A practical PC-Lab class is an essential part of the module. Students apply state-of-the-art machine learning techniques and understand how these are linked to standard econometrics. They command different machine learning methods and apply them to economic problems. They are aware of the respective advantages and shortcomings of these methods and discuss their results critically. |
Lecture Machine Learning in Econometrics, 8. Lesson
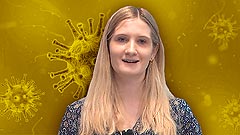

Title: | Lecture Machine Learning in Econometrics, 8. Lesson |
Description: | Vorlesung im SoSe 2021; Freitag, 07. Mai 2021 |
Creator: | Jantje Sönksen (author) |
Contributor: | ZDV Universität Tübingen (producer) |
Publisher: | ZDV Universität Tübingen |
Date Created: | 2021-05-07 |
Subjects: | Wirtschaftswissenschaft, Machine Learning, Econometrics, |
Identifier: | UT_20210507_004_sose21mleco_0001 |
Rights: | Rechtshinweise |
Abstracts: | This module illustrates how machine learning techniques can be exploited in economic research and applications. It offers a thorough analysis of a variety of tools in machine learning and links them to econometric analysis. The class focuses on supervised machine learning algorithms such as: decision trees, (logistic) regressions, naïve Bayes, nearest neighbor, neural networks, and support vector machines. The lecture also covers feature selection and hyper-parameter tuning methods. A practical PC-Lab class is an essential part of the module. Students apply state-of-the-art machine learning techniques and understand how these are linked to standard econometrics. They command different machine learning methods and apply them to economic problems. They are aware of the respective advantages and shortcomings of these methods and discuss their results critically. |
Lecture Machine Learning in Econometrics, 9. Lesson
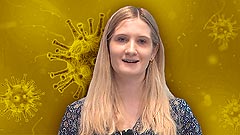

Title: | Lecture Machine Learning in Econometrics, 9. Lesson |
Description: | Vorlesung im SoSe 2021; Freitag, 14. Mai 2021 |
Creator: | Jantje Sönksen (author) |
Contributor: | ZDV Universität Tübingen (producer) |
Publisher: | ZDV Universität Tübingen |
Date Created: | 2021-05-14 |
Subjects: | Wirtschaftswissenschaft, Machine Learning, Econometrics, |
Identifier: | UT_20210514_001_sose21mleco_0001 |
Rights: | Rechtshinweise |
Abstracts: | This module illustrates how machine learning techniques can be exploited in economic research and applications. It offers a thorough analysis of a variety of tools in machine learning and links them to econometric analysis. The class focuses on supervised machine learning algorithms such as: decision trees, (logistic) regressions, naïve Bayes, nearest neighbor, neural networks, and support vector machines. The lecture also covers feature selection and hyper-parameter tuning methods. A practical PC-Lab class is an essential part of the module. Students apply state-of-the-art machine learning techniques and understand how these are linked to standard econometrics. They command different machine learning methods and apply them to economic problems. They are aware of the respective advantages and shortcomings of these methods and discuss their results critically. |
Lecture Machine Learning in Econometrics, 10. Lesson
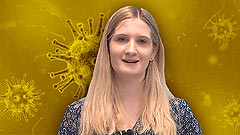

Title: | Lecture Machine Learning in Econometrics, 10. Lesson |
Description: | Vorlesung im SoSe 2021; Freitag, 14. Mai 2021 |
Creator: | Jantje Sönksen (author) |
Contributor: | ZDV Universität Tübingen (producer) |
Publisher: | ZDV Universität Tübingen |
Date Created: | 2021-05-14 |
Subjects: | Wirtschaftswissenschaft, Machine Learning, Econometrics, |
Identifier: | UT_20210514_002_sose21mleco_0001 |
Rights: | Rechtshinweise |
Abstracts: | This module illustrates how machine learning techniques can be exploited in economic research and applications. It offers a thorough analysis of a variety of tools in machine learning and links them to econometric analysis. The class focuses on supervised machine learning algorithms such as: decision trees, (logistic) regressions, naïve Bayes, nearest neighbor, neural networks, and support vector machines. The lecture also covers feature selection and hyper-parameter tuning methods. A practical PC-Lab class is an essential part of the module. Students apply state-of-the-art machine learning techniques and understand how these are linked to standard econometrics. They command different machine learning methods and apply them to economic problems. They are aware of the respective advantages and shortcomings of these methods and discuss their results critically. |
Lecture Machine Learning in Econometrics, 11. Lesson
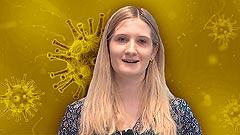

Title: | Lecture Machine Learning in Econometrics, 11. Lesson |
Description: | Vorlesung im SoSe 2021; Freitag, 14. Mai 2021 |
Creator: | Jantje Sönksen (author) |
Contributor: | ZDV Universität Tübingen (producer) |
Publisher: | ZDV Universität Tübingen |
Date Created: | 2021-05-14 |
Subjects: | Wirtschaftswissenschaft, Machine Learning, Econometrics, |
Identifier: | UT_20210514_003_sose21mleco_0001 |
Rights: | Rechtshinweise |
Abstracts: | This module illustrates how machine learning techniques can be exploited in economic research and applications. It offers a thorough analysis of a variety of tools in machine learning and links them to econometric analysis. The class focuses on supervised machine learning algorithms such as: decision trees, (logistic) regressions, naïve Bayes, nearest neighbor, neural networks, and support vector machines. The lecture also covers feature selection and hyper-parameter tuning methods. A practical PC-Lab class is an essential part of the module. Students apply state-of-the-art machine learning techniques and understand how these are linked to standard econometrics. They command different machine learning methods and apply them to economic problems. They are aware of the respective advantages and shortcomings of these methods and discuss their results critically. |
Lecture Machine Learning in Econometrics, 12. Lesson
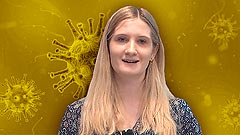

Title: | Lecture Machine Learning in Econometrics, 12. Lesson |
Description: | Vorlesung im SoSe 2021; Freitag, 14. Mai 2021 |
Creator: | Jantje Sönksen (author) |
Contributor: | ZDV Universität Tübingen (producer) |
Publisher: | ZDV Universität Tübingen |
Date Created: | 2021-05-14 |
Subjects: | Wirtschaftswissenschaft, Machine Learning, Econometrics, |
Identifier: | UT_20210514_004_sose21mleco_0001 |
Rights: | Rechtshinweise |
Abstracts: | This module illustrates how machine learning techniques can be exploited in economic research and applications. It offers a thorough analysis of a variety of tools in machine learning and links them to econometric analysis. The class focuses on supervised machine learning algorithms such as: decision trees, (logistic) regressions, naïve Bayes, nearest neighbor, neural networks, and support vector machines. The lecture also covers feature selection and hyper-parameter tuning methods. A practical PC-Lab class is an essential part of the module. Students apply state-of-the-art machine learning techniques and understand how these are linked to standard econometrics. They command different machine learning methods and apply them to economic problems. They are aware of the respective advantages and shortcomings of these methods and discuss their results critically. |
Lecture Machine Learning in Econometrics, 13. Lesson
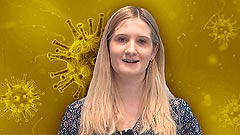

Title: | Lecture Machine Learning in Econometrics, 13. Lesson |
Description: | Vorlesung im SoSe 2021; Freitag, 21. Mai 2021 |
Creator: | Jantje Sönksen (author) |
Contributor: | ZDV Universität Tübingen (producer) |
Publisher: | ZDV Universität Tübingen |
Date Created: | 2021-05-21 |
Subjects: | Wirtschaftswissenschaft, Machine Learning, Econometrics, |
Identifier: | UT_20210521_001_sose21mleco_0001 |
Rights: | Rechtshinweise |
Abstracts: | This module illustrates how machine learning techniques can be exploited in economic research and applications. It offers a thorough analysis of a variety of tools in machine learning and links them to econometric analysis. The class focuses on supervised machine learning algorithms such as: decision trees, (logistic) regressions, naïve Bayes, nearest neighbor, neural networks, and support vector machines. The lecture also covers feature selection and hyper-parameter tuning methods. A practical PC-Lab class is an essential part of the module. Students apply state-of-the-art machine learning techniques and understand how these are linked to standard econometrics. They command different machine learning methods and apply them to economic problems. They are aware of the respective advantages and shortcomings of these methods and discuss their results critically. |
Lecture Machine Learning in Econometrics, 14. Lesson
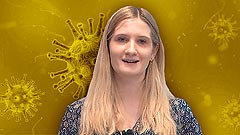

Title: | Lecture Machine Learning in Econometrics, 14. Lesson |
Description: | Vorlesung im SoSe 2021; Freitag, 21. Mai 2021 |
Creator: | Jantje Sönksen (author) |
Contributor: | ZDV Universität Tübingen (producer) |
Publisher: | ZDV Universität Tübingen |
Date Created: | 2021-05-21 |
Subjects: | Wirtschaftswissenschaft, Machine Learning, Econometrics, |
Identifier: | UT_20210521_002_sose21mleco_0001 |
Rights: | Rechtshinweise |
Abstracts: | This module illustrates how machine learning techniques can be exploited in economic research and applications. It offers a thorough analysis of a variety of tools in machine learning and links them to econometric analysis. The class focuses on supervised machine learning algorithms such as: decision trees, (logistic) regressions, naïve Bayes, nearest neighbor, neural networks, and support vector machines. The lecture also covers feature selection and hyper-parameter tuning methods. A practical PC-Lab class is an essential part of the module. Students apply state-of-the-art machine learning techniques and understand how these are linked to standard econometrics. They command different machine learning methods and apply them to economic problems. They are aware of the respective advantages and shortcomings of these methods and discuss their results critically. |
Lecture Machine Learning in Econometrics, 15. Lesson
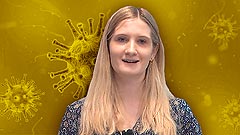

Title: | Lecture Machine Learning in Econometrics, 15. Lesson |
Description: | Vorlesung im SoSe 2021; Freitag, 21. Mai 2021 |
Creator: | Jantje Sönksen (author) |
Contributor: | ZDV Universität Tübingen (producer) |
Publisher: | ZDV Universität Tübingen |
Date Created: | 2021-05-21 |
Subjects: | Wirtschaftswissenschaft, Machine Learning, Econometrics, |
Identifier: | UT_20210521_003_sose21mleco_0001 |
Rights: | Rechtshinweise |
Abstracts: | This module illustrates how machine learning techniques can be exploited in economic research and applications. It offers a thorough analysis of a variety of tools in machine learning and links them to econometric analysis. The class focuses on supervised machine learning algorithms such as: decision trees, (logistic) regressions, naïve Bayes, nearest neighbor, neural networks, and support vector machines. The lecture also covers feature selection and hyper-parameter tuning methods. A practical PC-Lab class is an essential part of the module. Students apply state-of-the-art machine learning techniques and understand how these are linked to standard econometrics. They command different machine learning methods and apply them to economic problems. They are aware of the respective advantages and shortcomings of these methods and discuss their results critically. |
Lecture Machine Learning in Econometrics, 16. Lesson
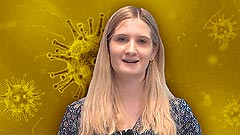

Title: | Lecture Machine Learning in Econometrics, 16. Lesson |
Description: | Vorlesung im SoSe 2021; Freitag, 21. Mai 2021 |
Creator: | Jantje Sönksen (author) |
Contributor: | ZDV Universität Tübingen (producer) |
Publisher: | ZDV Universität Tübingen |
Date Created: | 2021-05-21 |
Subjects: | Wirtschaftswissenschaft, Machine Learning, Econometrics, |
Identifier: | UT_20210521_004_sose21mleco_0001 |
Rights: | Rechtshinweise |
Abstracts: | This module illustrates how machine learning techniques can be exploited in economic research and applications. It offers a thorough analysis of a variety of tools in machine learning and links them to econometric analysis. The class focuses on supervised machine learning algorithms such as: decision trees, (logistic) regressions, naïve Bayes, nearest neighbor, neural networks, and support vector machines. The lecture also covers feature selection and hyper-parameter tuning methods. A practical PC-Lab class is an essential part of the module. Students apply state-of-the-art machine learning techniques and understand how these are linked to standard econometrics. They command different machine learning methods and apply them to economic problems. They are aware of the respective advantages and shortcomings of these methods and discuss their results critically. |
Lecture Machine Learning in Econometrics, 17. Lesson
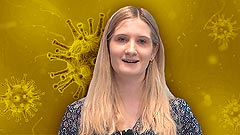

Title: | Lecture Machine Learning in Econometrics, 17. Lesson |
Description: | Vorlesung im SoSe 2021; Freitag, 04. Juni 2021 |
Creator: | Jantje Sönksen (author) |
Contributor: | ZDV Universität Tübingen (producer) |
Publisher: | ZDV Universität Tübingen |
Date Created: | 2021-06-04 |
Subjects: | Wirtschaftswissenschaft, Machine Learning, Econometrics, |
Identifier: | UT_20210604_001_sose21mleco_0001 |
Rights: | Rechtshinweise |
Abstracts: | This module illustrates how machine learning techniques can be exploited in economic research and applications. It offers a thorough analysis of a variety of tools in machine learning and links them to econometric analysis. The class focuses on supervised machine learning algorithms such as: decision trees, (logistic) regressions, naïve Bayes, nearest neighbor, neural networks, and support vector machines. The lecture also covers feature selection and hyper-parameter tuning methods. A practical PC-Lab class is an essential part of the module. Students apply state-of-the-art machine learning techniques and understand how these are linked to standard econometrics. They command different machine learning methods and apply them to economic problems. They are aware of the respective advantages and shortcomings of these methods and discuss their results critically. |
Lecture Machine Learning in Econometrics, 18. Lesson
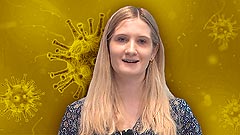

Title: | Lecture Machine Learning in Econometrics, 18. Lesson |
Description: | Vorlesung im SoSe 2021; Freitag, 04. Juni 2021 |
Creator: | Jantje Sönksen (author) |
Contributor: | ZDV Universität Tübingen (producer) |
Publisher: | ZDV Universität Tübingen |
Date Created: | 2021-06-04 |
Subjects: | Wirtschaftswissenschaft, Machine Learning, Econometrics, |
Identifier: | UT_20210604_002_sose21mleco_0001 |
Rights: | Rechtshinweise |
Abstracts: | This module illustrates how machine learning techniques can be exploited in economic research and applications. It offers a thorough analysis of a variety of tools in machine learning and links them to econometric analysis. The class focuses on supervised machine learning algorithms such as: decision trees, (logistic) regressions, naïve Bayes, nearest neighbor, neural networks, and support vector machines. The lecture also covers feature selection and hyper-parameter tuning methods. A practical PC-Lab class is an essential part of the module. Students apply state-of-the-art machine learning techniques and understand how these are linked to standard econometrics. They command different machine learning methods and apply them to economic problems. They are aware of the respective advantages and shortcomings of these methods and discuss their results critically. |
Lecture Machine Learning in Econometrics, 19. Lesson
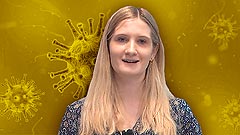

Title: | Lecture Machine Learning in Econometrics, 19. Lesson |
Description: | Vorlesung im SoSe 2021; Freitag, 04. Juni 2021 |
Creator: | Jantje Sönksen (author) |
Contributor: | ZDV Universität Tübingen (producer) |
Publisher: | ZDV Universität Tübingen |
Date Created: | 2021-06-04 |
Subjects: | Wirtschaftswissenschaft, Machine Learning, Econometrics, |
Identifier: | UT_20210604_003_sose21mleco_0001 |
Rights: | Rechtshinweise |
Abstracts: | This module illustrates how machine learning techniques can be exploited in economic research and applications. It offers a thorough analysis of a variety of tools in machine learning and links them to econometric analysis. The class focuses on supervised machine learning algorithms such as: decision trees, (logistic) regressions, naïve Bayes, nearest neighbor, neural networks, and support vector machines. The lecture also covers feature selection and hyper-parameter tuning methods. A practical PC-Lab class is an essential part of the module. Students apply state-of-the-art machine learning techniques and understand how these are linked to standard econometrics. They command different machine learning methods and apply them to economic problems. They are aware of the respective advantages and shortcomings of these methods and discuss their results critically. |
Lecture Machine Learning in Econometrics, 20. Lesson
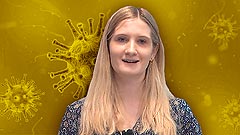

Title: | Lecture Machine Learning in Econometrics, 20. Lesson |
Description: | Vorlesung im SoSe 2021; Freitag, 04. Juni 2021 |
Creator: | Jantje Sönksen (author) |
Contributor: | ZDV Universität Tübingen (producer) |
Publisher: | ZDV Universität Tübingen |
Date Created: | 2021-06-04 |
Subjects: | Wirtschaftswissenschaft, Machine Learning, Econometrics, |
Identifier: | UT_20210604_004_sose21mleco_0001 |
Rights: | Rechtshinweise |
Abstracts: | This module illustrates how machine learning techniques can be exploited in economic research and applications. It offers a thorough analysis of a variety of tools in machine learning and links them to econometric analysis. The class focuses on supervised machine learning algorithms such as: decision trees, (logistic) regressions, naïve Bayes, nearest neighbor, neural networks, and support vector machines. The lecture also covers feature selection and hyper-parameter tuning methods. A practical PC-Lab class is an essential part of the module. Students apply state-of-the-art machine learning techniques and understand how these are linked to standard econometrics. They command different machine learning methods and apply them to economic problems. They are aware of the respective advantages and shortcomings of these methods and discuss their results critically. |
Lecture Machine Learning in Econometrics, 21. Lesson
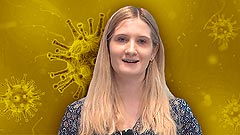

Title: | Lecture Machine Learning in Econometrics, 21. Lesson |
Description: | Vorlesung im SoSe 2021; Freitag, 11. Juni 2021 |
Creator: | Jantje Sönksen (author) |
Contributor: | ZDV Universität Tübingen (producer) |
Publisher: | ZDV Universität Tübingen |
Date Created: | 2021-06-11 |
Subjects: | Wirtschaftswissenschaft, Machine Learning, Econometrics, |
Identifier: | UT_20210611_001_sose21mleco_0001 |
Rights: | Rechtshinweise |
Abstracts: | This module illustrates how machine learning techniques can be exploited in economic research and applications. It offers a thorough analysis of a variety of tools in machine learning and links them to econometric analysis. The class focuses on supervised machine learning algorithms such as: decision trees, (logistic) regressions, naïve Bayes, nearest neighbor, neural networks, and support vector machines. The lecture also covers feature selection and hyper-parameter tuning methods. A practical PC-Lab class is an essential part of the module. Students apply state-of-the-art machine learning techniques and understand how these are linked to standard econometrics. They command different machine learning methods and apply them to economic problems. They are aware of the respective advantages and shortcomings of these methods and discuss their results critically. |
Lecture Machine Learning in Econometrics, 22. Lesson
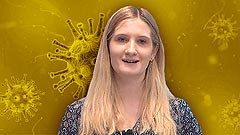

Title: | Lecture Machine Learning in Econometrics, 22. Lesson |
Description: | Vorlesung im SoSe 2021; Freitag, 11. Juni 2021 |
Creator: | Jantje Sönksen (author) |
Contributor: | ZDV Universität Tübingen (producer) |
Publisher: | ZDV Universität Tübingen |
Date Created: | 2021-06-11 |
Subjects: | Wirtschaftswissenschaft, Machine Learning, Econometrics, |
Identifier: | UT_20210611_002_sose21mleco_0001 |
Rights: | Rechtshinweise |
Abstracts: | This module illustrates how machine learning techniques can be exploited in economic research and applications. It offers a thorough analysis of a variety of tools in machine learning and links them to econometric analysis. The class focuses on supervised machine learning algorithms such as: decision trees, (logistic) regressions, naïve Bayes, nearest neighbor, neural networks, and support vector machines. The lecture also covers feature selection and hyper-parameter tuning methods. A practical PC-Lab class is an essential part of the module. Students apply state-of-the-art machine learning techniques and understand how these are linked to standard econometrics. They command different machine learning methods and apply them to economic problems. They are aware of the respective advantages and shortcomings of these methods and discuss their results critically. |
Lecture Machine Learning in Econometrics, 23. Lesson
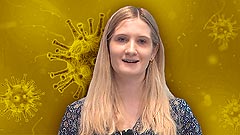

Title: | Lecture Machine Learning in Econometrics, 23. Lesson |
Description: | Vorlesung im SoSe 2021; Freitag, 11. Juni 2021 |
Creator: | Jantje Sönksen (author) |
Contributor: | ZDV Universität Tübingen (producer) |
Publisher: | ZDV Universität Tübingen |
Date Created: | 2021-06-11 |
Subjects: | Wirtschaftswissenschaft, Machine Learning, Econometrics, |
Identifier: | UT_20210611_003_sose21mleco_0001 |
Rights: | Rechtshinweise |
Abstracts: | This module illustrates how machine learning techniques can be exploited in economic research and applications. It offers a thorough analysis of a variety of tools in machine learning and links them to econometric analysis. The class focuses on supervised machine learning algorithms such as: decision trees, (logistic) regressions, naïve Bayes, nearest neighbor, neural networks, and support vector machines. The lecture also covers feature selection and hyper-parameter tuning methods. A practical PC-Lab class is an essential part of the module. Students apply state-of-the-art machine learning techniques and understand how these are linked to standard econometrics. They command different machine learning methods and apply them to economic problems. They are aware of the respective advantages and shortcomings of these methods and discuss their results critically. |
Lecture Machine Learning in Econometrics, 24. Lesson
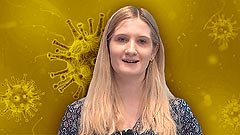

Title: | Lecture Machine Learning in Econometrics, 24. Lesson |
Description: | Vorlesung im SoSe 2021; Freitag, 11. Juni 2021 |
Creator: | Jantje Sönksen (author) |
Contributor: | ZDV Universität Tübingen (producer) |
Publisher: | ZDV Universität Tübingen |
Date Created: | 2021-06-11 |
Subjects: | Wirtschaftswissenschaft, Machine Learning, Econometrics, |
Identifier: | UT_20210611_004_sose21mleco_0001 |
Rights: | Rechtshinweise |
Abstracts: | This module illustrates how machine learning techniques can be exploited in economic research and applications. It offers a thorough analysis of a variety of tools in machine learning and links them to econometric analysis. The class focuses on supervised machine learning algorithms such as: decision trees, (logistic) regressions, naïve Bayes, nearest neighbor, neural networks, and support vector machines. The lecture also covers feature selection and hyper-parameter tuning methods. A practical PC-Lab class is an essential part of the module. Students apply state-of-the-art machine learning techniques and understand how these are linked to standard econometrics. They command different machine learning methods and apply them to economic problems. They are aware of the respective advantages and shortcomings of these methods and discuss their results critically. |
Lecture Machine Learning in Econometrics, 25. Lesson
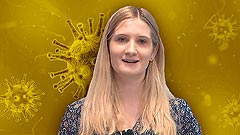

Title: | Lecture Machine Learning in Econometrics, 25. Lesson |
Description: | Vorlesung im SoSe 2021; Freitag, 25. Juni 2021 |
Creator: | Jantje Sönksen (author) |
Contributor: | ZDV Universität Tübingen (producer) |
Publisher: | ZDV Universität Tübingen |
Date Created: | 2021-06-25 |
Subjects: | Wirtschaftswissenschaft, Machine Learning, Econometrics, |
Identifier: | UT_20210625_001_sose21mleco_0001 |
Rights: | Rechtshinweise |
Abstracts: | This module illustrates how machine learning techniques can be exploited in economic research and applications. It offers a thorough analysis of a variety of tools in machine learning and links them to econometric analysis. The class focuses on supervised machine learning algorithms such as: decision trees, (logistic) regressions, naïve Bayes, nearest neighbor, neural networks, and support vector machines. The lecture also covers feature selection and hyper-parameter tuning methods. A practical PC-Lab class is an essential part of the module. Students apply state-of-the-art machine learning techniques and understand how these are linked to standard econometrics. They command different machine learning methods and apply them to economic problems. They are aware of the respective advantages and shortcomings of these methods and discuss their results critically. |
Lecture Machine Learning in Econometrics, 26. Lesson
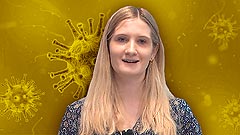

Title: | Lecture Machine Learning in Econometrics, 26. Lesson |
Description: | Vorlesung im SoSe 2021; Freitag, 25. Juni 2021 |
Creator: | Jantje Sönksen (author) |
Contributor: | ZDV Universität Tübingen (producer) |
Publisher: | ZDV Universität Tübingen |
Date Created: | 2021-06-25 |
Subjects: | Wirtschaftswissenschaft, Machine Learning, Econometrics, |
Identifier: | UT_20210625_002_sose21mleco_0001 |
Rights: | Rechtshinweise |
Abstracts: | This module illustrates how machine learning techniques can be exploited in economic research and applications. It offers a thorough analysis of a variety of tools in machine learning and links them to econometric analysis. The class focuses on supervised machine learning algorithms such as: decision trees, (logistic) regressions, naïve Bayes, nearest neighbor, neural networks, and support vector machines. The lecture also covers feature selection and hyper-parameter tuning methods. A practical PC-Lab class is an essential part of the module. Students apply state-of-the-art machine learning techniques and understand how these are linked to standard econometrics. They command different machine learning methods and apply them to economic problems. They are aware of the respective advantages and shortcomings of these methods and discuss their results critically. |
Lecture Machine Learning in Econometrics, 27. Lesson
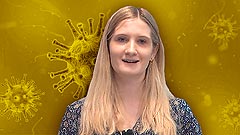

Title: | Lecture Machine Learning in Econometrics, 27. Lesson |
Description: | Vorlesung im SoSe 2021; Freitag, 25. Juni 2021 |
Creator: | Jantje Sönksen (author) |
Contributor: | ZDV Universität Tübingen (producer) |
Publisher: | ZDV Universität Tübingen |
Date Created: | 2021-06-25 |
Subjects: | Wirtschaftswissenschaft, Machine Learning, Econometrics, |
Identifier: | UT_20210625_003_sose21mleco_0001 |
Rights: | Rechtshinweise |
Abstracts: | This module illustrates how machine learning techniques can be exploited in economic research and applications. It offers a thorough analysis of a variety of tools in machine learning and links them to econometric analysis. The class focuses on supervised machine learning algorithms such as: decision trees, (logistic) regressions, naïve Bayes, nearest neighbor, neural networks, and support vector machines. The lecture also covers feature selection and hyper-parameter tuning methods. A practical PC-Lab class is an essential part of the module. Students apply state-of-the-art machine learning techniques and understand how these are linked to standard econometrics. They command different machine learning methods and apply them to economic problems. They are aware of the respective advantages and shortcomings of these methods and discuss their results critically. |
Lecture Machine Learning in Econometrics, 28. Lesson
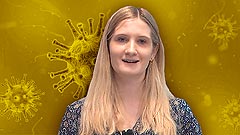

Title: | Lecture Machine Learning in Econometrics, 28. Lesson |
Description: | Vorlesung im SoSe 2021; Freitag, 25. Juni 2021 |
Creator: | Jantje Sönksen (author) |
Contributor: | ZDV Universität Tübingen (producer) |
Publisher: | ZDV Universität Tübingen |
Date Created: | 2021-06-25 |
Subjects: | Wirtschaftswissenschaft, Machine Learning, Econometrics, |
Identifier: | UT_20210625_004_sose21mleco_0001 |
Rights: | Rechtshinweise |
Abstracts: | This module illustrates how machine learning techniques can be exploited in economic research and applications. It offers a thorough analysis of a variety of tools in machine learning and links them to econometric analysis. The class focuses on supervised machine learning algorithms such as: decision trees, (logistic) regressions, naïve Bayes, nearest neighbor, neural networks, and support vector machines. The lecture also covers feature selection and hyper-parameter tuning methods. A practical PC-Lab class is an essential part of the module. Students apply state-of-the-art machine learning techniques and understand how these are linked to standard econometrics. They command different machine learning methods and apply them to economic problems. They are aware of the respective advantages and shortcomings of these methods and discuss their results critically. |
Lecture Machine Learning in Econometrics, 29. Lesson
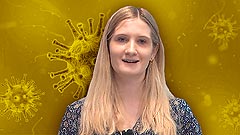

Title: | Lecture Machine Learning in Econometrics, 29. Lesson |
Description: | Vorlesung im SoSe 2021; Mittwoch, 30. Juni 2021 |
Creator: | Jantje Sönksen (author) |
Contributor: | ZDV Universität Tübingen (producer) |
Publisher: | ZDV Universität Tübingen |
Date Created: | 2021-06-30 |
Subjects: | Wirtschaftswissenschaft, Machine Learning, Econometrics, |
Identifier: | UT_20210630_001_sose21mleco_0001 |
Rights: | Rechtshinweise |
Abstracts: | This module illustrates how machine learning techniques can be exploited in economic research and applications. It offers a thorough analysis of a variety of tools in machine learning and links them to econometric analysis. The class focuses on supervised machine learning algorithms such as: decision trees, (logistic) regressions, naïve Bayes, nearest neighbor, neural networks, and support vector machines. The lecture also covers feature selection and hyper-parameter tuning methods. A practical PC-Lab class is an essential part of the module. Students apply state-of-the-art machine learning techniques and understand how these are linked to standard econometrics. They command different machine learning methods and apply them to economic problems. They are aware of the respective advantages and shortcomings of these methods and discuss their results critically. |
Lecture Machine Learning in Econometrics, 30. Lesson
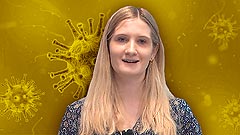

Title: | Lecture Machine Learning in Econometrics, 30. Lesson |
Description: | Vorlesung im SoSe 2021; Mittwoch, 30. Juni 2021 |
Creator: | Jantje Sönksen (author) |
Contributor: | ZDV Universität Tübingen (producer) |
Publisher: | ZDV Universität Tübingen |
Date Created: | 2021-06-30 |
Subjects: | Wirtschaftswissenschaft, Machine Learning, Econometrics, |
Identifier: | UT_20210630_002_sose21mleco_0001 |
Rights: | Rechtshinweise |
Abstracts: | This module illustrates how machine learning techniques can be exploited in economic research and applications. It offers a thorough analysis of a variety of tools in machine learning and links them to econometric analysis. The class focuses on supervised machine learning algorithms such as: decision trees, (logistic) regressions, naïve Bayes, nearest neighbor, neural networks, and support vector machines. The lecture also covers feature selection and hyper-parameter tuning methods. A practical PC-Lab class is an essential part of the module. Students apply state-of-the-art machine learning techniques and understand how these are linked to standard econometrics. They command different machine learning methods and apply them to economic problems. They are aware of the respective advantages and shortcomings of these methods and discuss their results critically. |
Lecture Machine Learning in Econometrics, 31. Lesson
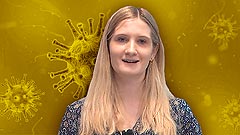

Title: | Lecture Machine Learning in Econometrics, 31. Lesson |
Description: | Vorlesung im SoSe 2021; Freitag, 02. Juli 2021 |
Creator: | Jantje Sönksen (author) |
Contributor: | ZDV Universität Tübingen (producer) |
Publisher: | ZDV Universität Tübingen |
Date Created: | 2021-07-02 |
Subjects: | Wirtschaftswissenschaft, Machine Learning, Econometrics, |
Identifier: | UT_20210702_001_sose21mleco_0001 |
Rights: | Rechtshinweise |
Abstracts: | This module illustrates how machine learning techniques can be exploited in economic research and applications. It offers a thorough analysis of a variety of tools in machine learning and links them to econometric analysis. The class focuses on supervised machine learning algorithms such as: decision trees, (logistic) regressions, naïve Bayes, nearest neighbor, neural networks, and support vector machines. The lecture also covers feature selection and hyper-parameter tuning methods. A practical PC-Lab class is an essential part of the module. Students apply state-of-the-art machine learning techniques and understand how these are linked to standard econometrics. They command different machine learning methods and apply them to economic problems. They are aware of the respective advantages and shortcomings of these methods and discuss their results critically. |
Lecture Machine Learning in Econometrics, 32. Lesson
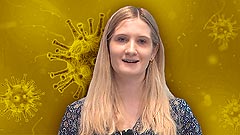

Title: | Lecture Machine Learning in Econometrics, 32. Lesson |
Description: | Vorlesung im SoSe 2021; Freitag, 02. Juli 2021 |
Creator: | Jantje Sönksen (author) |
Contributor: | ZDV Universität Tübingen (producer) |
Publisher: | ZDV Universität Tübingen |
Date Created: | 2021-07-02 |
Subjects: | Wirtschaftswissenschaft, Machine Learning, Econometrics, |
Identifier: | UT_20210702_002_sose21mleco_0001 |
Rights: | Rechtshinweise |
Abstracts: | This module illustrates how machine learning techniques can be exploited in economic research and applications. It offers a thorough analysis of a variety of tools in machine learning and links them to econometric analysis. The class focuses on supervised machine learning algorithms such as: decision trees, (logistic) regressions, naïve Bayes, nearest neighbor, neural networks, and support vector machines. The lecture also covers feature selection and hyper-parameter tuning methods. A practical PC-Lab class is an essential part of the module. Students apply state-of-the-art machine learning techniques and understand how these are linked to standard econometrics. They command different machine learning methods and apply them to economic problems. They are aware of the respective advantages and shortcomings of these methods and discuss their results critically. |
Lecture Machine Learning in Econometrics, 33. Lesson
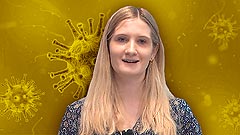

Title: | Lecture Machine Learning in Econometrics, 33. Lesson |
Description: | Vorlesung im SoSe 2021; Freitag, 02. Juli 2021 |
Creator: | Jantje Sönksen (author) |
Contributor: | ZDV Universität Tübingen (producer) |
Publisher: | ZDV Universität Tübingen |
Date Created: | 2021-07-02 |
Subjects: | Wirtschaftswissenschaft, Machine Learning, Econometrics, |
Identifier: | UT_20210702_003_sose21mleco_0001 |
Rights: | Rechtshinweise |
Abstracts: | This module illustrates how machine learning techniques can be exploited in economic research and applications. It offers a thorough analysis of a variety of tools in machine learning and links them to econometric analysis. The class focuses on supervised machine learning algorithms such as: decision trees, (logistic) regressions, naïve Bayes, nearest neighbor, neural networks, and support vector machines. The lecture also covers feature selection and hyper-parameter tuning methods. A practical PC-Lab class is an essential part of the module. Students apply state-of-the-art machine learning techniques and understand how these are linked to standard econometrics. They command different machine learning methods and apply them to economic problems. They are aware of the respective advantages and shortcomings of these methods and discuss their results critically. |
Lecture Machine Learning in Econometrics, 34. Lesson
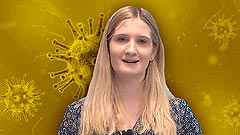

Title: | Lecture Machine Learning in Econometrics, 34. Lesson |
Description: | Vorlesung im SoSe 2021; Freitag, 02. Juli 2021 |
Creator: | Jantje Sönksen (author) |
Contributor: | ZDV Universität Tübingen (producer) |
Publisher: | ZDV Universität Tübingen |
Date Created: | 2021-07-02 |
Subjects: | Wirtschaftswissenschaft, Machine Learning, Econometrics, |
Identifier: | UT_20210702_004_sose21mleco_0001 |
Rights: | Rechtshinweise |
Abstracts: | This module illustrates how machine learning techniques can be exploited in economic research and applications. It offers a thorough analysis of a variety of tools in machine learning and links them to econometric analysis. The class focuses on supervised machine learning algorithms such as: decision trees, (logistic) regressions, naïve Bayes, nearest neighbor, neural networks, and support vector machines. The lecture also covers feature selection and hyper-parameter tuning methods. A practical PC-Lab class is an essential part of the module. Students apply state-of-the-art machine learning techniques and understand how these are linked to standard econometrics. They command different machine learning methods and apply them to economic problems. They are aware of the respective advantages and shortcomings of these methods and discuss their results critically. |
Lecture Machine Learning in Econometrics, 35. Lesson
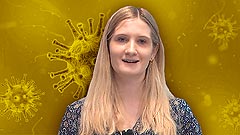

Title: | Lecture Machine Learning in Econometrics, 35. Lesson |
Description: | Vorlesung im SoSe 2021; Freitag, 09. Juli 2021 |
Creator: | Jantje Sönksen (author) |
Contributor: | ZDV Universität Tübingen (producer) |
Publisher: | ZDV Universität Tübingen |
Date Created: | 2021-07-09 |
Subjects: | Wirtschaftswissenschaft, Machine Learning, Econometrics, |
Identifier: | UT_20210709_001_sose21mleco_0001 |
Rights: | Rechtshinweise |
Abstracts: | This module illustrates how machine learning techniques can be exploited in economic research and applications. It offers a thorough analysis of a variety of tools in machine learning and links them to econometric analysis. The class focuses on supervised machine learning algorithms such as: decision trees, (logistic) regressions, naïve Bayes, nearest neighbor, neural networks, and support vector machines. The lecture also covers feature selection and hyper-parameter tuning methods. A practical PC-Lab class is an essential part of the module. Students apply state-of-the-art machine learning techniques and understand how these are linked to standard econometrics. They command different machine learning methods and apply them to economic problems. They are aware of the respective advantages and shortcomings of these methods and discuss their results critically. |
Lecture Machine Learning in Econometrics, 36. Lesson
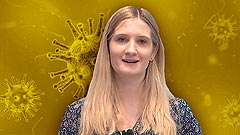

Title: | Lecture Machine Learning in Econometrics, 36. Lesson |
Description: | Vorlesung im SoSe 2021; Freitag, 09. Juli 2021 |
Creator: | Jantje Sönksen (author) |
Contributor: | ZDV Universität Tübingen (producer) |
Publisher: | ZDV Universität Tübingen |
Date Created: | 2021-07-09 |
Subjects: | Wirtschaftswissenschaft, Machine Learning, Econometrics, |
Identifier: | UT_20210709_002_sose21mleco_0001 |
Rights: | Rechtshinweise |
Abstracts: | This module illustrates how machine learning techniques can be exploited in economic research and applications. It offers a thorough analysis of a variety of tools in machine learning and links them to econometric analysis. The class focuses on supervised machine learning algorithms such as: decision trees, (logistic) regressions, naïve Bayes, nearest neighbor, neural networks, and support vector machines. The lecture also covers feature selection and hyper-parameter tuning methods. A practical PC-Lab class is an essential part of the module. Students apply state-of-the-art machine learning techniques and understand how these are linked to standard econometrics. They command different machine learning methods and apply them to economic problems. They are aware of the respective advantages and shortcomings of these methods and discuss their results critically. |
Lecture Machine Learning in Econometrics, 37. Lesson
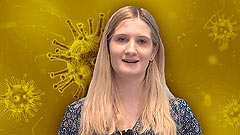

Title: | Lecture Machine Learning in Econometrics, 37. Lesson |
Description: | Vorlesung im SoSe 2021; Freitag, 09. Juli 2021 |
Creator: | Jantje Sönksen (author) |
Contributor: | ZDV Universität Tübingen (producer) |
Publisher: | ZDV Universität Tübingen |
Date Created: | 2021-07-09 |
Subjects: | Wirtschaftswissenschaft, Machine Learning, Econometrics, |
Identifier: | UT_20210709_003_sose21mleco_0001 |
Rights: | Rechtshinweise |
Abstracts: | This module illustrates how machine learning techniques can be exploited in economic research and applications. It offers a thorough analysis of a variety of tools in machine learning and links them to econometric analysis. The class focuses on supervised machine learning algorithms such as: decision trees, (logistic) regressions, naïve Bayes, nearest neighbor, neural networks, and support vector machines. The lecture also covers feature selection and hyper-parameter tuning methods. A practical PC-Lab class is an essential part of the module. Students apply state-of-the-art machine learning techniques and understand how these are linked to standard econometrics. They command different machine learning methods and apply them to economic problems. They are aware of the respective advantages and shortcomings of these methods and discuss their results critically. |
Lecture Machine Learning in Econometrics, 38. Lesson
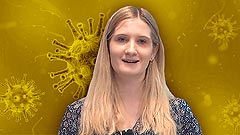

Title: | Lecture Machine Learning in Econometrics, 38. Lesson |
Description: | Vorlesung im SoSe 2021; Freitag, 09. Juli 2021 |
Creator: | Jantje Sönksen (author) |
Contributor: | ZDV Universität Tübingen (producer) |
Publisher: | ZDV Universität Tübingen |
Date Created: | 2021-07-09 |
Subjects: | Wirtschaftswissenschaft, Machine Learning, Econometrics, |
Identifier: | UT_20210709_004_sose21mleco_0001 |
Rights: | Rechtshinweise |
Abstracts: | This module illustrates how machine learning techniques can be exploited in economic research and applications. It offers a thorough analysis of a variety of tools in machine learning and links them to econometric analysis. The class focuses on supervised machine learning algorithms such as: decision trees, (logistic) regressions, naïve Bayes, nearest neighbor, neural networks, and support vector machines. The lecture also covers feature selection and hyper-parameter tuning methods. A practical PC-Lab class is an essential part of the module. Students apply state-of-the-art machine learning techniques and understand how these are linked to standard econometrics. They command different machine learning methods and apply them to economic problems. They are aware of the respective advantages and shortcomings of these methods and discuss their results critically. |
Lecture Machine Learning in Econometrics, 39. Lesson
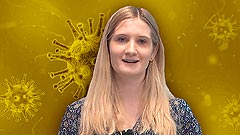

Title: | Lecture Machine Learning in Econometrics, 39. Lesson |
Description: | Vorlesung im SoSe 2021; Freitag, 16. Juli 2021 |
Creator: | Jantje Sönksen (author) |
Contributor: | ZDV Universität Tübingen (producer) |
Publisher: | ZDV Universität Tübingen |
Date Created: | 2021-07-16 |
Subjects: | Wirtschaftswissenschaft, Machine Learning, Econometrics, |
Identifier: | UT_20210716_001_sose21mleco_0001 |
Rights: | Rechtshinweise |
Abstracts: | This module illustrates how machine learning techniques can be exploited in economic research and applications. It offers a thorough analysis of a variety of tools in machine learning and links them to econometric analysis. The class focuses on supervised machine learning algorithms such as: decision trees, (logistic) regressions, naïve Bayes, nearest neighbor, neural networks, and support vector machines. The lecture also covers feature selection and hyper-parameter tuning methods. A practical PC-Lab class is an essential part of the module. Students apply state-of-the-art machine learning techniques and understand how these are linked to standard econometrics. They command different machine learning methods and apply them to economic problems. They are aware of the respective advantages and shortcomings of these methods and discuss their results critically. |
Lecture Machine Learning in Econometrics, 40. Lesson
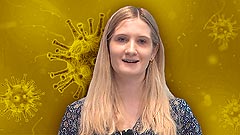

Title: | Lecture Machine Learning in Econometrics, 40. Lesson |
Description: | Vorlesung im SoSe 2021; Freitag, 16. Juli 2021 |
Creator: | Jantje Sönksen (author) |
Contributor: | ZDV Universität Tübingen (producer) |
Publisher: | ZDV Universität Tübingen |
Date Created: | 2021-07-16 |
Subjects: | Wirtschaftswissenschaft, Machine Learning, Econometrics, |
Identifier: | UT_20210716_002_sose21mleco_0001 |
Rights: | Rechtshinweise |
Abstracts: | This module illustrates how machine learning techniques can be exploited in economic research and applications. It offers a thorough analysis of a variety of tools in machine learning and links them to econometric analysis. The class focuses on supervised machine learning algorithms such as: decision trees, (logistic) regressions, naïve Bayes, nearest neighbor, neural networks, and support vector machines. The lecture also covers feature selection and hyper-parameter tuning methods. A practical PC-Lab class is an essential part of the module. Students apply state-of-the-art machine learning techniques and understand how these are linked to standard econometrics. They command different machine learning methods and apply them to economic problems. They are aware of the respective advantages and shortcomings of these methods and discuss their results critically. |
Lecture Machine Learning in Econometrics, 41. Lesson
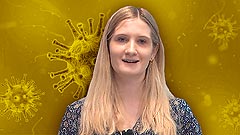

Title: | Lecture Machine Learning in Econometrics, 41. Lesson |
Description: | Vorlesung im SoSe 2021; Freitag, 16. Juli 2021 |
Creator: | Jantje Sönksen (author) |
Contributor: | ZDV Universität Tübingen (producer) |
Publisher: | ZDV Universität Tübingen |
Date Created: | 2021-07-16 |
Subjects: | Wirtschaftswissenschaft, Machine Learning, Econometrics, |
Identifier: | UT_20210716_003_sose21mleco_0001 |
Rights: | Rechtshinweise |
Abstracts: | This module illustrates how machine learning techniques can be exploited in economic research and applications. It offers a thorough analysis of a variety of tools in machine learning and links them to econometric analysis. The class focuses on supervised machine learning algorithms such as: decision trees, (logistic) regressions, naïve Bayes, nearest neighbor, neural networks, and support vector machines. The lecture also covers feature selection and hyper-parameter tuning methods. A practical PC-Lab class is an essential part of the module. Students apply state-of-the-art machine learning techniques and understand how these are linked to standard econometrics. They command different machine learning methods and apply them to economic problems. They are aware of the respective advantages and shortcomings of these methods and discuss their results critically. |
Lecture Machine Learning in Econometrics, 42. Lesson
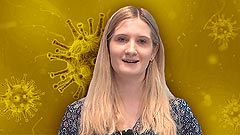

Title: | Lecture Machine Learning in Econometrics, 42. Lesson |
Description: | Vorlesung im SoSe 2021; Freitag, 16. Juli 2021 |
Creator: | Jantje Sönksen (author) |
Contributor: | ZDV Universität Tübingen (producer) |
Publisher: | ZDV Universität Tübingen |
Date Created: | 2021-07-16 |
Subjects: | Wirtschaftswissenschaft, Machine Learning, Econometrics, |
Identifier: | UT_20210716_004_sose21mleco_0001 |
Rights: | Rechtshinweise |
Abstracts: | This module illustrates how machine learning techniques can be exploited in economic research and applications. It offers a thorough analysis of a variety of tools in machine learning and links them to econometric analysis. The class focuses on supervised machine learning algorithms such as: decision trees, (logistic) regressions, naïve Bayes, nearest neighbor, neural networks, and support vector machines. The lecture also covers feature selection and hyper-parameter tuning methods. A practical PC-Lab class is an essential part of the module. Students apply state-of-the-art machine learning techniques and understand how these are linked to standard econometrics. They command different machine learning methods and apply them to economic problems. They are aware of the respective advantages and shortcomings of these methods and discuss their results critically. |
Lecture Machine Learning in Econometrics, 43. Lesson
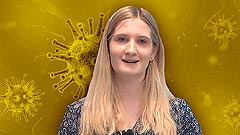

Title: | Lecture Machine Learning in Econometrics, 43. Lesson |
Description: | Vorlesung im SoSe 2021; Freitag, 23. Juli 2021 |
Creator: | Jantje Sönksen (author) |
Contributor: | ZDV Universität Tübingen (producer) |
Publisher: | ZDV Universität Tübingen |
Date Created: | 2021-07-23 |
Subjects: | Wirtschaftswissenschaft, Machine Learning, Econometrics, |
Identifier: | UT_20210723_001_sose21mleco_0001 |
Rights: | Rechtshinweise |
Abstracts: | This module illustrates how machine learning techniques can be exploited in economic research and applications. It offers a thorough analysis of a variety of tools in machine learning and links them to econometric analysis. The class focuses on supervised machine learning algorithms such as: decision trees, (logistic) regressions, naïve Bayes, nearest neighbor, neural networks, and support vector machines. The lecture also covers feature selection and hyper-parameter tuning methods. A practical PC-Lab class is an essential part of the module. Students apply state-of-the-art machine learning techniques and understand how these are linked to standard econometrics. They command different machine learning methods and apply them to economic problems. They are aware of the respective advantages and shortcomings of these methods and discuss their results critically. |
Lecture Machine Learning in Econometrics, 44. Lesson
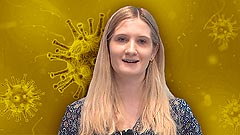

Title: | Lecture Machine Learning in Econometrics, 44. Lesson |
Description: | Vorlesung im SoSe 2021; Freitag, 23. Juli 2021 |
Creator: | Jantje Sönksen (author) |
Contributor: | ZDV Universität Tübingen (producer) |
Publisher: | ZDV Universität Tübingen |
Date Created: | 2021-07-23 |
Subjects: | Wirtschaftswissenschaft, Machine Learning, Econometrics, |
Identifier: | UT_20210723_002_sose21mleco_0001 |
Rights: | Rechtshinweise |
Abstracts: | This module illustrates how machine learning techniques can be exploited in economic research and applications. It offers a thorough analysis of a variety of tools in machine learning and links them to econometric analysis. The class focuses on supervised machine learning algorithms such as: decision trees, (logistic) regressions, naïve Bayes, nearest neighbor, neural networks, and support vector machines. The lecture also covers feature selection and hyper-parameter tuning methods. A practical PC-Lab class is an essential part of the module. Students apply state-of-the-art machine learning techniques and understand how these are linked to standard econometrics. They command different machine learning methods and apply them to economic problems. They are aware of the respective advantages and shortcomings of these methods and discuss their results critically. |